
Blind face restoration [8, 2, 3] refers to the process of achieving a high quality, sharp and noise free facial image from an input that is low quality, blurry and noisy. The main aim of blind face restoration methods is to generate a visually pleasing version of the original image while maintaining the facial structure of the person. In contrast, face retrieval systems [6, 7] use as input a query image and faces in the search dataset that are matching with the query face are given as results. This is performed by comparing the query face with every existing face in the dataset using a face recognition algorithm [9, 1, 4] that can generate a similarity score between two faces. The similarity score is then compared to a predefined threshold in order to predict if the faces belong to the same person or not and the faces that are predicted to belong to the same person as the query image are given as matching results. There are many factors that can negatively impact the performance of a face recognition method such as facial pose, age, lighting conditions, occlusion, and quality of the images in terms of sharpness, noise and color.
In this work, we investigate if the negative impacts of low quality of historical images on face retrieval performance can be alleviated by applying blind face restoration prior to running facial retrieval. The experiments were conducted on historical images dating back to the World War II era [5]. This digital archive contains around 160 000 photos, of which only little over 800 are colored photos. Faces in many of the photographs are blurry, noisy, and mostly black and white. The ability to remove these deteriorations from historical photos, would potentially enhance the face retrieval performance on them. We selected for our experiments a subset of 442 faces, including 53 faces of five famous historical figures (Carl Gustaf Emil Mannerheim, Johan Wilhelm Rangell, Adolf Hitler, Prince Gustaf Adolf, and Risto Ryti), which were used as query images. High quality images of these five historical figures that were not included in the dataset were used for querying.
Two different versions of a state-of-the-art blind face restoration method were applied on the face dataset. The first version aims to restore the faces in terms of sharpness and noise, while the other version also colorizes the images in addition to performing the same restoration as the first version. This resulted in three different versions of the same dataset: original version, where the quality is low, an upscaled version without colorization, and an upscaled version with colorization. The query images of the historical figures were then used separately in the three versions of the face dataset to find matching images by using face recognition. This way, three different sets of results were obtained, one for each version of the dataset. The results showed that upscaling and colorization of the faces resulted in a very minor boost in finding more matching images. However, it was observed that applying blind face restoration resulted in a significantly fewer wrong matches (faces belonging to different people that were wrongly predicted as belonging to the same person). The upscaled and colorized version of the dataset had the least amount of wrong matches, followed by the upscaled and non-colorized version, and finally the original version. In other words, applying blind face restoration did not help in finding more images of the query person that were missed before due to low quality, but it helped greatly in removing the false results.
In conclusion, we explored and analyzed the positive impacts of blind face restoration on face retrieval. We observed better face retrieval performance in a series of experiments where we applied different face restoration methods on historical low-quality images. The significant reduction in the number of false face matches by using face restoration can greatly improve the usability of the results of a face retrieval applied on a large dataset.
References
[1] Anil K Jain and Stan Z Li. Handbook of face recognition. Vol. 1. Springer,2011.
[2] Xiaoming Li et al. “Blind face restoration via deep multi-scale component dictionaries”. In: European Conference on Computer Vision. Springer.2020, pp. 399–415.
[3] Yehong Liao and Xueyin Lin. “Blind image restoration with eigen-face sub-space”. In: IEEE Transactions on Image Processing 14.11 (2005), pp. 1766-1772.
[4] Omkar M. Parkhi, Andrea Vedaldi, and Andrew Zisserman. “Deep Face Recognition”. In: Proceedings of the British Machine Vision Conference
(BMVC). Ed. by Mark W. Jones Xianghua Xie and Gary K. L. Tam. BMVA Press, Sept. 2015, pp. 41.1–41.12.
[5] SA-kuva: Finnish Wartime Photograph Archive. Finland: The Finnish Defence Forces. url: http://sa-kuva.fi/.
[6] Jinhui Tang, Zechao Li, and Xiang Zhu. “Supervised deep hashing for scalable face image retrieval”. In: Pattern Recognition 75 (2018). Distance Metric Learning for Pattern Recognition, pp. 25–32.
[7] Ahmad S Tarawneh et al. “Deep face image retrieval: A comparative study with dictionary learning”. In: 2019 10th International Conference on Information and Communication Systems (ICICS). IEEE. 2019, pp. 185–192.
[8] Xintao Wang et al. “Towards real-world blind face restoration with generative facial prior”. In: Proceedings of the IEEE/CVF Conference on Computer Vision and Pattern Recognition. 2021, pp. 9168–9178.
[9] Wenyi Zhao et al. “Face recognition: A literature survey”. In: ACM com- puting surveys (CSUR) 35.4 (2003), pp. 399–458.
Mert Seker received his MSc. degree from Tampere University, Finland in 2021 majoring in Data Engineering & Machine Learning. He is currently work- ing as a researcher at Tampere University, working on interdisciplinary projects involving the application of state-of-the-art computer vision and machine learn- ing methods on photographic studies. His main fields of studies are computer vision and machine learning.
Back
SPONSORS
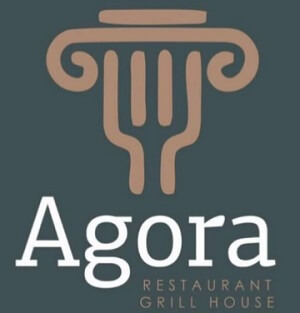
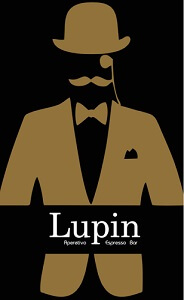